Ouster 3D lidar is powering autonomy. Lidar accurately captures and monitors environments in extreme detail, enabling everything from autonomous last-mile delivery rovers, to unmanned aerial systems, to autonomous vehicles to perceive and react to the world around them.
While lidar is a critical component of any vision and sensing system, there is often a gap between sensing the data and making sense of the cumulative data. Most autonomous machines need to understand their position and orientation within their environments in order to navigate safely and efficiently. While there are open source Simultaneous Localization and Mapping (SLAM) solutions available, getting them tightly integrated into applications and viable for commercial purposes can be a multi-year endeavor. Given the rapid pace of innovation, even multiple months is far too long.
This is why in collaboration with Kudan, we are introducing a SLAM evaluation program that makes this process simple and free for Ouster’s customers. With Kudan Lidar SLAM (KdLidar), customers get access to a free commercial-ready SLAM software along with guided support, enabling them to take their applications to market faster and with higher confidence.
In this post, we’ll discuss the role of SLAM for applications and a guide to effectively evaluating SLAM for your projects so you can get started quickly.
We also have a webinar with Kudan you can view: Zero to SLAM in 30 Minutes
What is SLAM?
Simultaneous Localization and Mapping (SLAM) is a method that gives machines the ability to understand their position and orientation within an environment. SLAM gives machines spatial awareness by sensing, creating, and constantly updating a representation of the world around them.
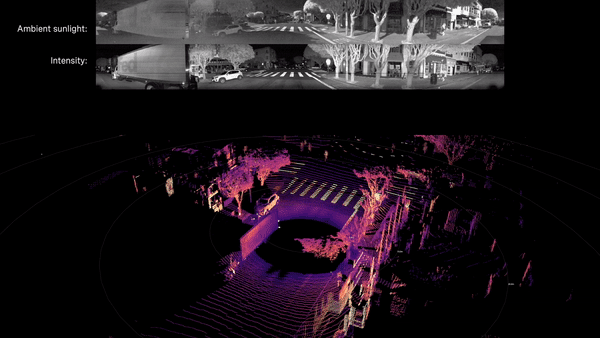
Most humans can do this well without much effort, but trying to get a machine to do this is another matter. Just as people will recognize landmarks and road features to create a mental map of a place they visit, SLAM creates a point cloud representation of a map from the detected lidar points and uses this point cloud map to determine the lidar sensor’s position at any given time. SLAM will use just enough of the unique features it detects to create the point cloud.
Do I need SLAM for my project?
Location, Location, Location
The primary driver for whether you need SLAM for your project is directly related to how important it is for your device to know its location and position within a space.
Some examples of applications where SLAM can be critical include:
- Autonomous mobile robots and last mile delivery vehicles that need to navigate in unpredictable external environments, including dodging pedestrians and other moving vehicles
- Autonomous vehicles that need to navigate safely and efficiently from Point A to Point B
- Mapping and surveying vehicles, robots, or drones, particularly within indoor and GPS-denied areas such as urban canyons, underground mines or covered structures. SLAM can continue to provide precise position data in these scenarios
In short, SLAM is valuable (and often critical) for most autonomy projects.
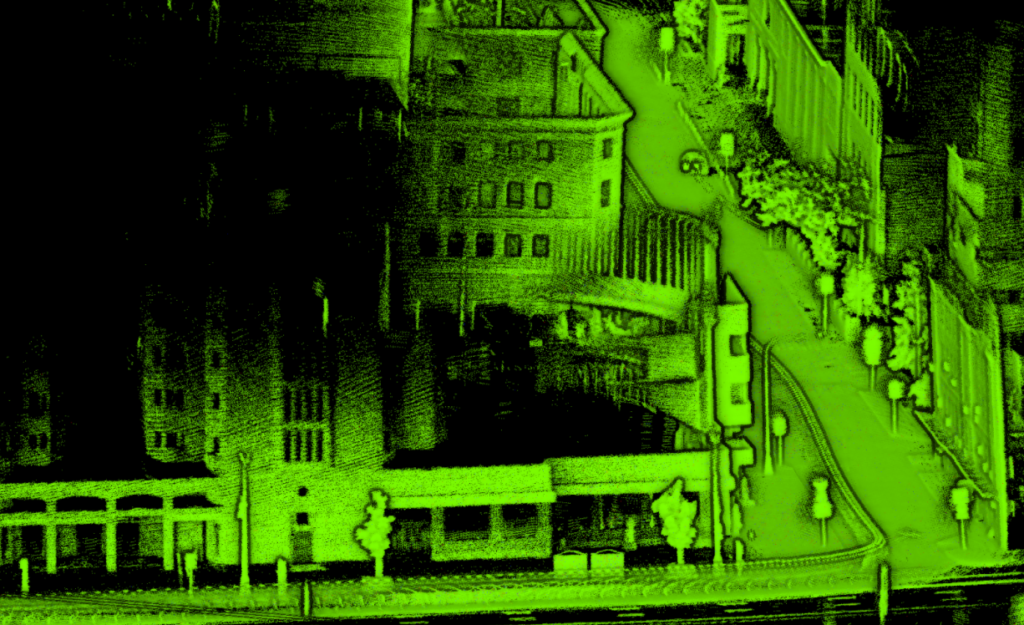
Guide to evaluating SLAM
In this section, we’ll cover the key elements to consider when evaluating a SLAM solution.
The four elements
While each project is unique, there are several elements that are core to evaluating a SLAM solution for your project.
- Accuracy: How accurate is the point cloud map against the real world?
- Repeatability accuracy: How accurately can the system position itself within a known map?
- Relocalization: How well and accurately does the system recognize the position on the map?
- Resources: Does everything work within the available computing and memory resources?
Accuracy: How accurately does the point cloud represent the real world?
Ouster’s high-resolution lidar generates very accurate measurements and our point clouds generate similarly accurate measurements. However, the reality is that all sensor data tends to drift without correction, and errors will accumulate over time. SLAM algorithms can compensate for this via mechanisms like Loop Closure and integration of other sensor data, but you should expect some deviation in any single map point of up to 5cm given that lidar sensor depth accuracy already has 2-3 cm error.
However, during the evaluation process, you should evaluate the general completeness of the map. Does the map capture the boundaries, obstacles, and fixtures correctly? Deviation is typically under 10 cm at any given point without fusing any other sensors. Fine-tuning and fusing other sensors such as GNSS and IMU brings the error significantly lower. Fine-tuning the mapping capabilities of the system will come during the actual development of the system. Also, you might want to consider the accuracy impact of real-time processing or post-processing depending on your applications.
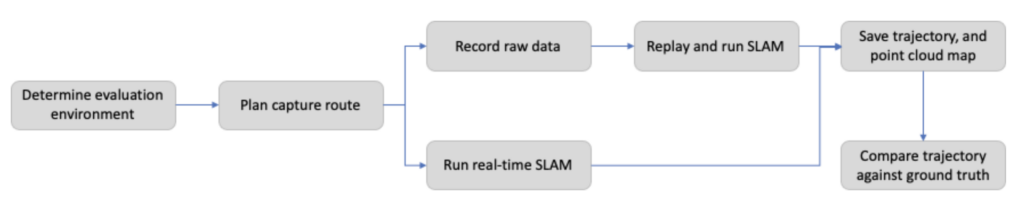
At an early stage, especially as you set up your system and tweak parameters, you may opt to use a recorded dataset to see how the different parameters affect the system performance. As you get closer to an integrated commercial product, you will likely test the system using real-time mode to ensure robust operations in different conditions.
It’s good practice to build (and keep updated) a suite of recorded data that represents normal and corner cases of operations that are important to the success of your product, and to use it as part of your regression suite and validation process as development continues on the product.
Repeatability: How accurately can the system position itself within a known map?
The other type of accuracy, especially important for robotics use cases, is a measurement of how precise or repeatable tracking of the device is. Many applications involve navigating to specific spots and routines repeatedly over time. You want the device to be able to precisely repeat these activities, whether it’s maneuvering on streets or aisles in a warehouse. Repeatability measures how accurately a device positions itself against the point cloud map once it is created. Even without optimization, you should expect repeatability accuracy to be very high, with commercial deployments seeing sub-cm accuracy for repeatability.

Relocalization: How well and accurately does the system recognize its position on the map?
Relocalization is the capability for a machine to scan its current surroundings to figure out where it is, without having any prior information about its position. This is important if SLAM loses track of features around it, or when the machine is first turned on or reset.
Your positioning system needs to be robust enough to continue its operation despite gaps in past trajectory and location data. One of the easiest methods to test this is to “kidnap” the device – blindfold it and move it to some new location, and see if it can figure out where it is.

The device should be able to determine its current location after “kidnapping” and continue operating from its new position. Areas of failure may indicate the need to capture more data in the area to enhance the map, or you may need to introduce routines and safe movements to reestablish tracking.
Getting started
Previously, evaluating and integrating SLAM solutions was a complicated, lengthy process.
In collaboration with Kudan, we’re excited to introduce Kudan Lidar SLAM (KdLidar), a SLAM evaluation kit for Ouster lidar. KdLidar is a quick and easy way to evaluate commercial-quality SLAM to see if it’s suitable for your projects. KdLidar works across different platforms and is available via a comprehensive API library. The evaluation software will be provided as a ROS node encapsulating SLAM features, such as mapping and tracking. The required computing environment is Ubuntu 18.04+ running ROS Melodic.
After the free evaluation phase, customers can move to the development phase with Kudan SLAM. This will open more complex features such as tighter sensor integration (IMU / INS / GNSS / Wheel Odometry) for more accurate and robust tracking and advanced map management features (dense point cloud generation, map merging, map splitting, automatically aligning multiple maps from different sessions and map streaming), and a full API library.
Ready to get started? Request access to the free evaluation software, quick start documentation, and tips on running the evaluation. We also have a webinar (Zero to SLAM in 30 minutes) for you to watch.
Happy SLAMming!