Computer vision and machine learning are essential to most engineering teams that use lidar. At Ouster, Reyhaneh Kazerani is a Machine Learning Engineer working on computer vision projects with data labeling and annotation. In an interview with Re-Work Deep Learning, she explains the implications of Ouster’s unique data output and her perspective on future machine learning developments.
Ouster develops high-resolution, affordable multi-beam flash lidar sensors for applications including drones, mapping, robotics, and autonomous vehicles. But in addition to building the hardware, we’re also working on challenges in perception using our lidar, and engineers like Reyhaneh are at the forefront of this rapidly expanding field.
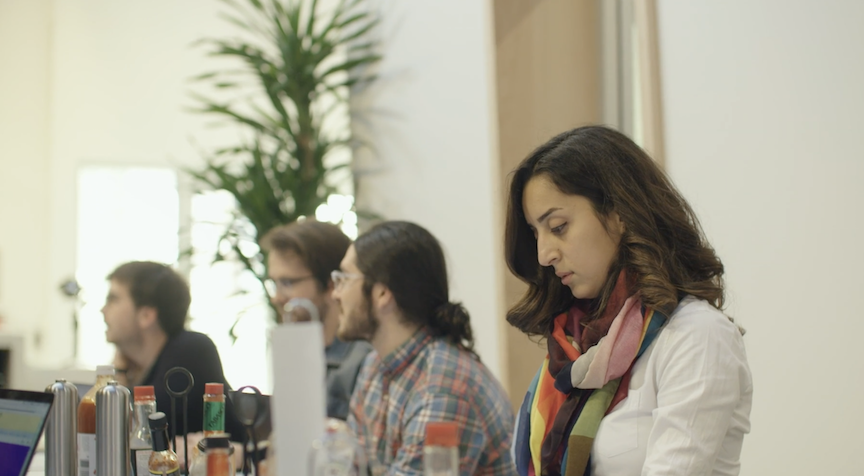
1. What makes Ouster different in the world of machine learning?
AI and machine learning is having a transformative impact on robotics in numerous ways. While these technologies are still in their infancy, their rapid growth requires low-cost, robust sensors more than ever. To most of these applications, lidar has been the central sensor. Most available lidars are quite expensive, and not robust for different conditions. Ouster’s lidar is not only low-cost and accessible to researchers and engineers but also high quality and high performance.
2. How does this structured lidar data impact customers? What’s the value that Ouster brings?
Lidar data has incredible benefits – rich spatial information and lighting agnostic sensing to name a few – but it lacks the raw resolution and efficient array structure of camera images, and 3D point clouds are still more difficult to encode in a neural net or process with hardware acceleration. With the tradeoffs between both sensing modalities in mind, we set out to bring the best aspects of lidar and camera together in a single device from the very beginning. The OS1 now outputs fixed resolution ambient images, signal images, and depth images in real time, all without a camera.
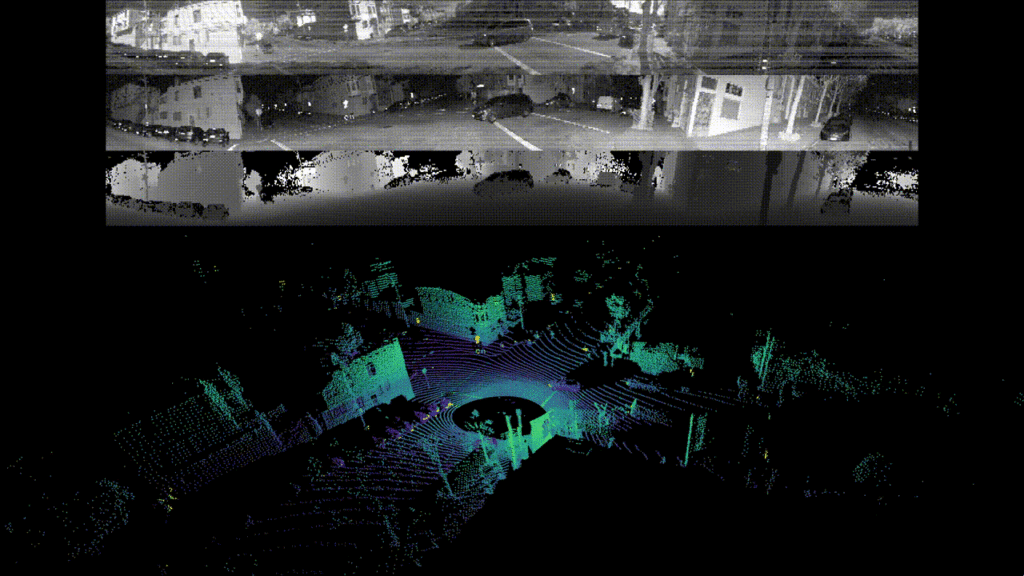
While RGB-D cameras and traditional flash lidar sensors are also capable of outputting structured range data, neither class of sensors has comparable range, range resolution, field of view, or robustness in outdoor environments, compared to the Ouster OS1 lidar sensor. However, these shorter-range structured 3D cameras can still benefit from the work we’re doing, and we encourage manufacturers of these products to consider our approach.
3. What are some of the main bottlenecks in productivity that Ouster’s structured lidar output will solve?
Convolutional neural networks have recently pushed the limits in computer vision by using inherent grid structure in images. Unstructured lidar data has made it difficult to use these convolutional neural networks. The majority of the current approaches are using preprocessing steps to project the unstructured data into a grid structure. However, the OS1 sensor outputs fixed resolution image frames with depth, signal, and ambient data at each pixel; we’re able to feed these images directly into deep learning algorithms that were battle tested and developed to work with the structured data produced by cameras. We get the best of both worlds by having the advantages of both 3D and 2D approaches without any sensor fusion or preprocessing.
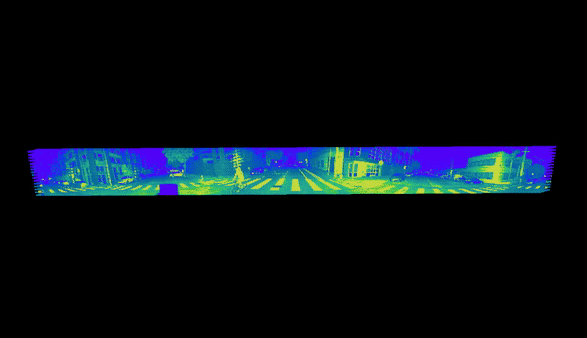
Using this unique capability, we’ve worked with our labeling partners to take advantage of our structured data in their labeling tools in order to minimize the cost of labeling, increase their capabilities, and improve the accuracy of the annotation significantly.
4. What developments in machine learning are you most excited for, and how is Ouster going to be a part of that?
I believe there is a lot of unexplored potential in machine learning. Deep learning has outrun the state of art in many areas and has been a far first runner in many tasks. These improvements in architecture, algorithms and models, have been pushing the machine learning limits but these are all impossible without having the abundance of useful and labeled data. Ouster has a mission of producing reliable, rugged, high resolution and low cost sensors that outputs unique data to unlock the new improvements in computer vision and perception.
Besides our machine learning achievements, Ouster continues to improve with innovative technology to produce better sensors that pave the way for research.
Originally published for the Re-Work Deep Learning blog.