In January 2022, Ouster joined transportation leaders at the Transportation Research Board Annual Meeting to exchange ideas for making the future of mobility safer, more efficient, and more sustainable. As part of this event, Ouster co-sponsored the 2022 Transportation Forecasting Competition (TRANSFOR22) with the Center of Urban Informatics and Progress at The University of Tennessee at Chattanooga, NSF, City of Chattanooga, and Seoul Robotics.
TRANSFOR22 focused on addressing vulnerable road user safety and called for innovative approaches to this challenge. Over 1.3 million lives continue to be lost every year due to traffic-related accidents, with vulnerable road users being the majority of these numbers. Disabled and elderly pedestrians are at a particularly heightened risk.
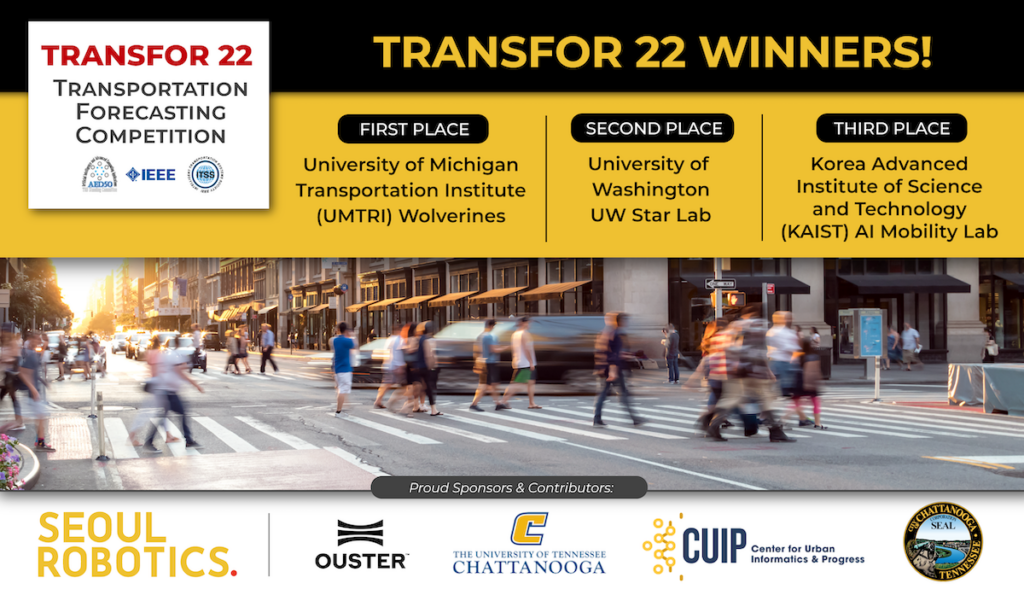
In this post, we’ll be featuring the incredible finalist projects that came out of TRANSFOR22. Please reach out if you’re interested in learning more or collaborating with Ouster on your projects!
The TRANSFOR22 challenge
The goal of this year’s TRANSFOR22 challenge was to encourage innovative solutions to improving vulnerable road user safety. Participants were given signal performance logs (SPM) from a traffic controller and a corresponding labeled lidar dataset collected from the MLK Smart Corridor in Chattanooga, Tennessee. They were then challenged to evaluate the data accuracy of vulnerable pedestrian groups, predict the time needed for them to safely cross a street, and develop solutions for dynamic signal control.
The lidar data was pre-collected by Ouster digital lidar sensors and processed with Seoul Robotics’ 3D perception software to provide 15,440 unique objects labeled as car, pedestrian, cyclist, or other. The lidar dataset also included per-object XYZ position, speed, velocity, and trajectory.
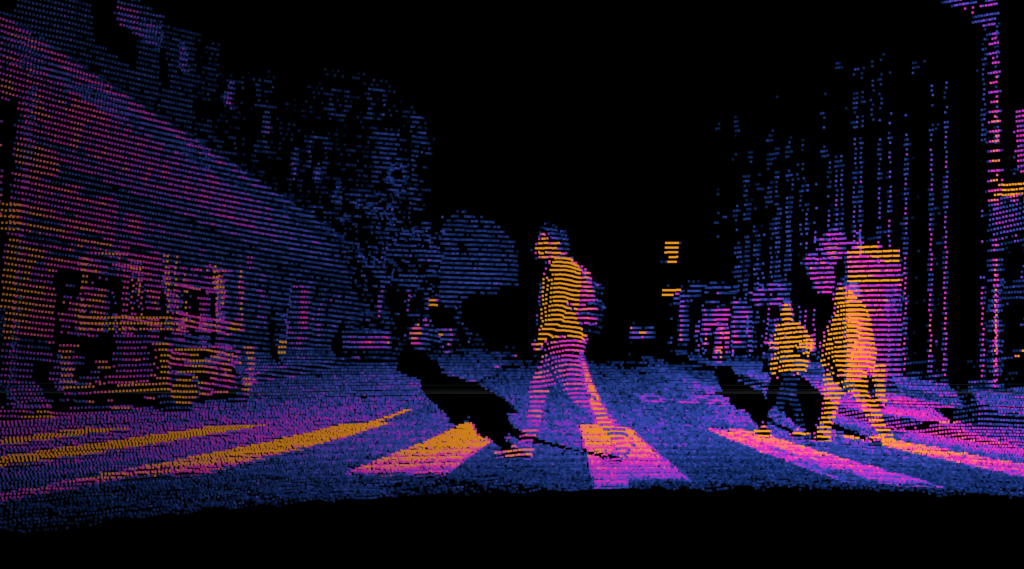
Teams presented their projects during the TRB Annual Meeting in Washington, D.C. Final rankings were determined based on classification accuracy, novelty of solutions, quality of code, quality of paper and presentations.
Huge congratulations to the three finalist teams: University of Michigan, University of Washington, and Korea Advanced Institute of Science and Technology (KAIST)!
University of Michigan, Transportation Research Institute
UMTRI is a leading global research center whose mission is to advance safe, equitable, and efficient transportation and mobility.
For this challenge, the UMTRI team developed novel methods of classifying and predicting pedestrians’ crossing risk and crossing times at intersections. In the real world, such models can be used for dynamic signal timing. Congratulations to the UMTRI team for winning first place at TRANSFOR22!
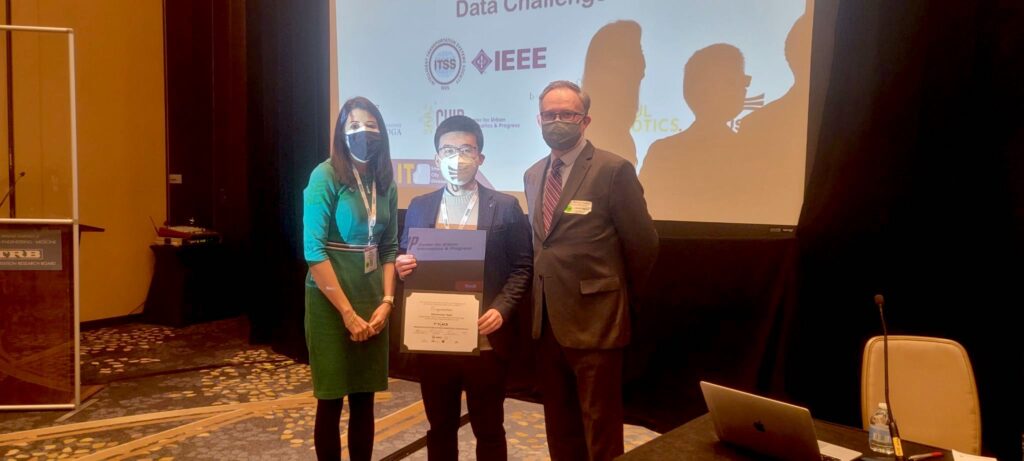
Shown in photo: Dr. Mina Sartipi (Center for Urban Informatics and Progress at the University of Chattanooga – Tennessee), UMTRI team member, and Steven Sheffield (Business Development, ITS at Ouster)
The team decided to augment the standard time-to-collision calculation with their own “trajectory-following-TTC” (time-to-collision) approach between pedestrians and vehicles to quantify the pedestrian risk of crossing the street at any given time. This “trajectory-following-TTC” model calculates the TTC using robust metrics from previously sampled trajectories, instead of using an object’s current path. This approach made the risk calculation more robust to measurement noise and took into consideration that headings of vehicles can change drastically in just seconds in urban intersections.
The team used deep learning models to predict the amount of time a pedestrian would require to cross the street considering both pedestrian-based and signal-based features. With these, the UMTRI team was most accurately able to determine the amount of time required for a pedestrian to cross and whether the traffic signal allotted enough time to do so safely.
University of Washington, STAR Lab
Founded in 2003, Smart Transportation Applications and Research (STAR) Lab combines multidisciplinary expertise to research and develop ITS theories and applications.
For this challenge, the STAR Lab team developed an early detection algorithm that could alert a traffic signal early on if a pedestrian required more time based on their walking speed. The team utilized per-object position, velocity, and trajectory to filter out non-pedestrian data, identified “higher-risk” pedestrians by clustering velocity time series, and finally sub-classified them against general pedestrians based on object shapes.
Instead of predicting crossing time, the team predicted average crossing speed of pedestrians after defining and constructing features from a three-second early detection window.
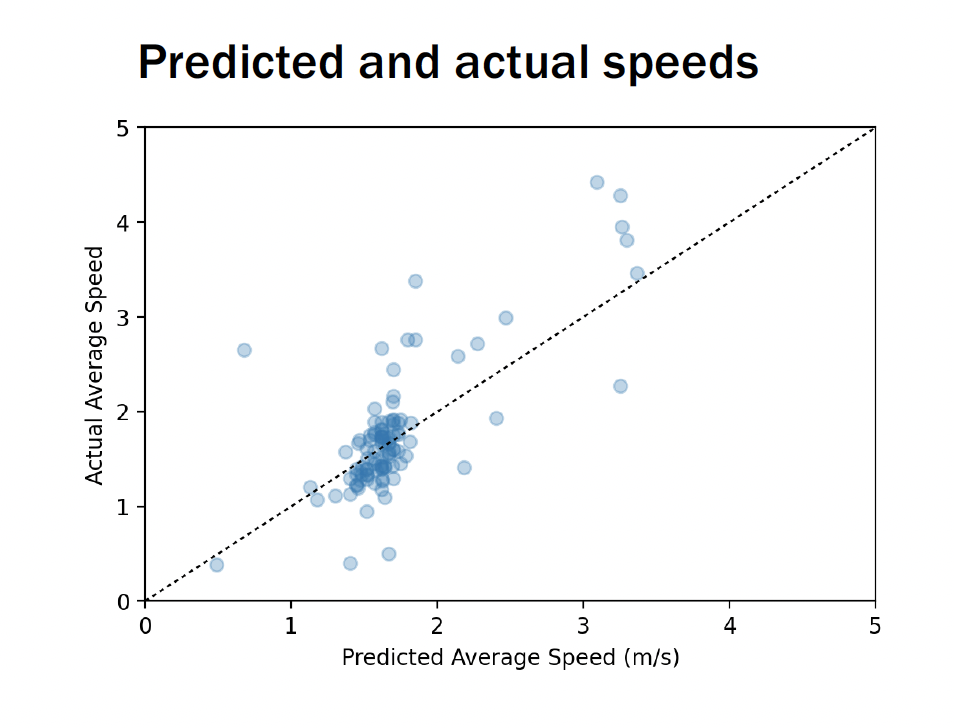
Congratulations to the STAR Lab team for winning second place at TRANSFOR22!
Korea Advanced Institute of Science and Technology (KAIST), AI x Mobility Lab
AIxMobility lab at KAIST specializes in the design, operations, and management methodologies of transportation systems for sustainable society using intelligent transportation systems combining cutting edge Artificial Intelligence Technologies.
For this challenge, the AIxMobility Lab team created an arrival-time prediction model that predicted the time needed for a “pedestrian on foot” and “pedestrian in a wheelchair” to cross the street. Using machine learning algorithms, the team first sub-classified pedestrians as “pedestrian on foot” versus “pedestrian in a wheelchair” based on object motion and geometry information . They achieved 93% classification accuracy.
To create the arrival-time prediction model, the team tested Deep Neural Networks (DNN), Long Short Term Memory (LSTM), Gated Recurrent Unit (GRU), and Transformer to accurately predict pedestrians’ arrival time and obtain a result with a 1.2 seconds error margin.
Congratulations to the KAIST team for winning third place at TRANSFOR22!
TRANSFOR22: big step towards road safety
TRANSFOR22 would not have been possible without AED50 Artificial Intelligence and Advanced Computing Applications, the organizers of this program, and the IEEE ITSS Technical Activities Sub-Committee “Smart Mobility and Transportation 5.0”. And of course, without all the participating teams and winning finalists.
Making quantifiable progress requires better insights on road user patterns that can inform proactive safety measures, dynamic traffic systems, and equitable mobility options, just to name a few. The first step is data that is accurate, robust, and accounts for all types of road users, all types of weather conditions, and all hours of the day.
Ouster’s mission is to help achieve safer, more efficient mobility with our digital lidar sensors. We’re proud to have been part of TRB and TRANSFOR22, and to see the incredible progress all the teams made in just one day. Achieving Vision Zero and a safe future requires tight private-public-academic partnerships just like this one.
Interested in partnering with Ouster? Learn more about Ouster’s ITS solutions or reach out to one of our industry experts!